About this tool
FL-DTD is a tool to identify the targets of novel compounds in a tissue-specific manner. We built this pipeline based on a hypothesis about existence of feedback loops after compound perturbation. In detail, normal cells are under a stable status, which are usually maintained as dynamic protein interaction networks. When external stimulus take impacts on any node of a network, it is expected to spread its effects to nearby nodes and finally eliminate such effects of external stimulus in form of feedback loops so that to avoid toxicity to the whole cell. We hypothesize that if drug perturbation altered the activity of target proteins, it will also induce some network responses, e.g. the inhibitor of a node protein may activate expression of upstream nodes and repress expression of downstream nodes, which results in deviation of node interaction
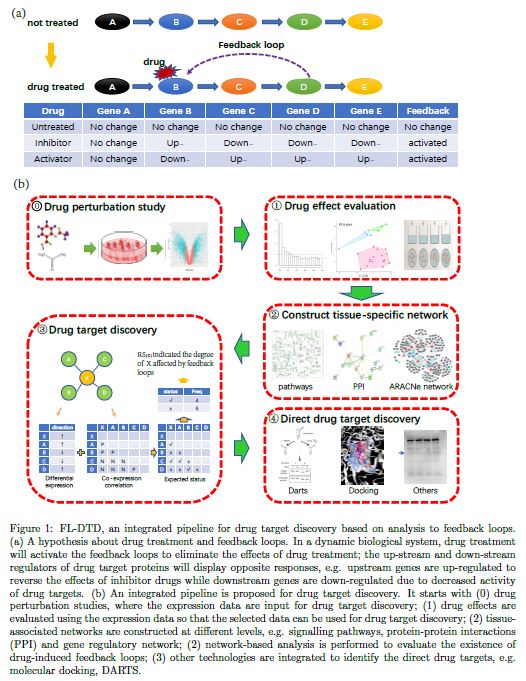
Contact
If you have any problem to use this tool, please contact menggf @ gmail.com
Citation
Dong Lu, Rongrong Pan, Wenxuan Wu, Yanyan Zhang, Shensuo Li, Hong Xu, Jialan Huang, Jianhua Xia, Qun Wang, Xin Luan, Chao Lv, Weidong Zhang, Guofeng Meng, FL-DTD: an integrated pipeline to predict the drug interacting targets by feedback loop-based network analysis, Briefings in Bioinformatics, 2022;, bbac263, https://doi.org/10.1093/bib/bbac263
Begin analysis..
Gene ranking scores
Help information
When to use this tool
1. To predict the tissue-specific targets of novel compounds
FLD-TD can predict the drug interacting targets in a tissue-specific manner. It takes the differential expression analysis results of drug perturbation data as input and ouput the direct and indirect binding target of novel compounds.
2. To screen the compounds targeted to some protein
With accumulation of drug perturbation data, e.g. Connectivity Map, it is possible to screen the compounds with perturbation activity to some protein, e.g. STAT3. In this way, FLD-TD can predict the compound with best activity to target proteins.
How to use this tool
1. prepare the differential expression result of drug perturbation
The input file stores a data frame with at least three column: Gene.symbol, P.Value, logFC. The accepted file format is "*.csv" seperated by comma with header
2. Selected the right cell types
We built the reference networks using 34 TCGA RNA-seq data, including two BRCA subtypes, ER+ and ER-). Therefore, users can choose one of cancer types according to the cell line used drug perturbation study.
3. Set the parameters
Currently, there are only two parameter setting. 'Max. DEG. pval' is the p-value cutoff of differential expression results. If no enough DEGs was input, FLD-TD will not report any prediction and raise a error. 'Min. coexp. correlation (r)' is the cutoff to filter the refenece network. High r cutoff can lead to good confidence but bad sensitivity.
4. Understand the outcomes
FLD-TD reports the ranks, e.g. R1, R2, R3, R4 and R5, of each gene according to response score of feedback loops. Here, five networks are used to calculate the response scores and the ranks may reflect the different properties of drug MOA.
The 'Rank' column reports the minimum ranks among five networks.